Blog Machine Learning In Cybersecurity
Complicated attack techniques need equally sophisticated countervailing forces. Companies that have been the victims of an increase in hacking attempts are now employing machine learning to detect attack patterns and respond proactively. Machine learning-driven autonomous threat detection and mitigation systems can detect and mitigate threats in real-time and with minimal human intervention.
Integrating machine learning with existing security procedures reduces expenses, improves response time, and helps chart sound mitigation strategies. Here's why investing in machine learning to enhance your cybersecurity makes sense.
What is machine learning in cybersecurity?
Machine learning is a branch of artificial intelligence (AI) that uses data to generate or analyze expert-level knowledge. The data is either mined or gathered through internet connection testing to build an expert-level model. This model helps identify risks and strengthen cybersecurity defences.
Machine learning helps identify vulnerabilities in software systems and remediate them before an attack occurs. Machine learning helps predict risks based on past performance and create a remediation plan when trained adequately. Machine learning can be connected to cybersecurity using a data science team called a Cybersecurity Analytics team (CSA). The team collects and analyzes data, simulates scenarios, and makes inferences to predict vulnerabilities. The CSA is the last line of defence that uses the data to formulate action plans.
How is machine learning bringing down cybersecurity costs?
Artificial intelligence and machine learning reduce the labour needed to detect and respond to cyber threats, making them cost-effective solutions. According to Capgemini's analysis, the average cost reduction is 12%, with some companies saving more than 15%.
Machine learning can predict and remediate risks before attackers can exploit them. Machine learning anticipates potential fallout by simulating scenarios to identify risk areas and perform high-level assessments. For example, a company may use machine learning to identify social engineering attacks, user accounts with stolen credentials, and other risks.
Machine learning can also be used to prepare for, and respond to, attacks. For example, an IT department may use machine learning to create a security posture review to help organizations prepare for attacks.
In conjunction with data mining, machine learning can analyze vast volumes of data in seconds, i.e., considerably faster than humans. Further, they can apply fixes and mitigate risks in near real-time, significantly reducing the response time. The ability of attackers to quickly gain access to an organization’s infrastructure makes razor-sharp detection and reaction critical.
How can machine learning help organizations?
According to the Identity Theft Research Center, 2021 was a record-breaking year in the United States, with the number of data breaches by the end of the third quarter exceeding all of 2020 by 17%. Analysts can use machine learning to improve various security procedures. For security analysts, machine learning simplifies discovering new cyberattacks to comprehend previous cyber-attacks better and develop related defences.
Here are some ways AI and machine learning can assist enterprises with cybersecurity:
Artificial intelligence and machine learning combine behavioural analysis and constantly evolving parameters to detect anomalies that could indicate an attack.
Through artificial intelligence and machine learning, cyber risks can be predicted and remediated before attackers can exploit them.
Artificial intelligence and machine learning can detect cyber threats and automatically build protective patches without human involvement.
Machine learning takes over repetitive tasks like triaging intelligence and monitoring network log analysis to free up resources.
Can machine learning replace expert manual penetration testing?
The short answer is no. Similar to a vulnerability scan or VA Scan, machines and automated scans can only pick up so much. Although VA scans are valuable tools to help stay on top of the security of your environment regularly, it should be understood that they come with limitations. Sometimes low-risk findings in a VA scan are identified as high-risk in a pentest. This is because a pentest explores your environment from an attacker’s perspective. It separates the noise and outlines the most critical findings that require remediation, and explains why.
A Pentest, on the other hand, is an active analysis of your systems to look for both known and unknown vulnerabilities. This is done by simulating a real-world attack on your systems and then trying to exploit any vulnerabilities that are found. A pentest can be run internally or externally, depending on the nature of the engagement. The Packetlabs team testing involves 5% automated testing techniques and 95% manual testing.
Key Takeaway
While the power of machine learning in bolstering cybersecurity cannot be overstated, it is not the magic pill to cure all cyber maladies. Although AI and ML are improving rapidly, technology is only as good as the analysts using them. Packetlabs’ deep knowledge can help analysts gain insight into their organization’s security components. Our penetration testing abilities can reveal the weak points in the system and offer actionable advisories on merging machine learning with existing security protocols to strengthen their posture.
Featured Posts
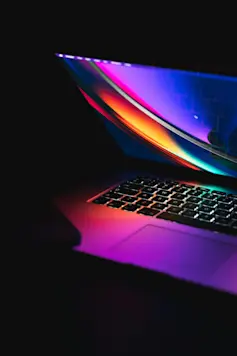
July 07 - Blog
Are You Using WPA3?
Discover how WPA3 strengthens Wi-Fi security, with enhanced protection against password cracking, encrypted public networks, and improved privacy.
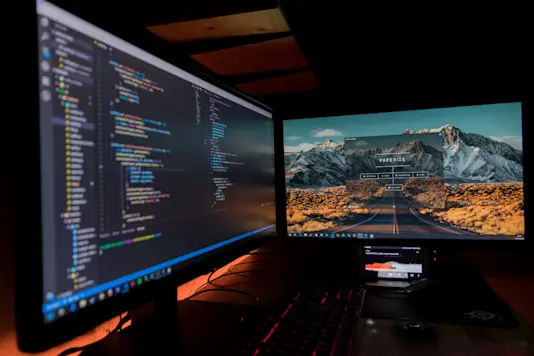
July 02 - Blog
Blackwood APT Uses AiTM Attacks to Target Software Updates
Blackwood APT uses AiTM attacks that are set to target software updates. Is your organization prepared? Learn more in today's blog.
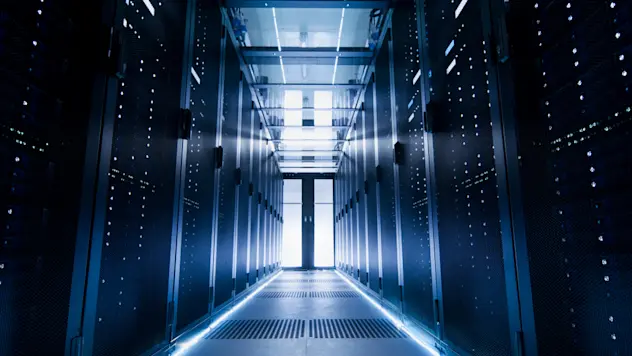
June 12 - Blog
What is an Initial Access Broker?
What is an initial access broker? With the emergence of Ransomware as a Service, operators often rely on initial access brokers to obtain an initial foothold on the network. Learn more today.